Hi everyone, Malena here—I’m an investigative reporter at The Markup, but I’m taking a break from my usual deep diving to talk about something a little more airy and light: birds!
More specifically, I’ve become interested in how artificial intelligence (AI) is reshaping the world of ornithology. One of the most substantial ways is through BirdCast, a project that uses machine learning to help scientists predict how birds migrate based on weather data.
BirdCast is a collaboration between the Cornell Lab of Ornithology, Colorado State University, and UMass Amherst. Launched in 2018, it uses data collected from 143 radar sites across the lower 48 U.S. states to identify bird movements and forecast how those birds will migrate.
Andrew Farnsworth is a visiting scientist at the Cornell Lab of Ornithology who has worked on the project since its inception in the 1990s and wrote the grant for what would become BirdCast’s current iteration. The following interview has been edited for clarity and length.
Malena: How did you get into birding?
Andrew: I had a grandfather who was a scientist. He was a biochemist, but he had binoculars at the house and he had at the time what was sort of considered like the cutting edge materials in a field guide. Coupled with being in a relatively green place for a suburb of New York City, in Westchester County [it] all sort of came together as the spark. [The] wood duck and downy woodpecker were kind of the gateways.
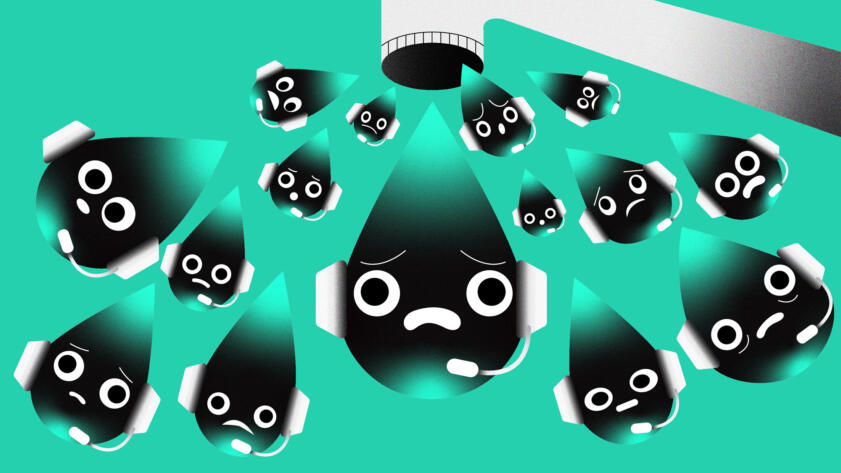
Hello World
The Secret Water Footprint of AI Technology
A conversation with Shaolei Ren
Malena: Why is it important to understand bird migration?
Andrew: There are three good, reasonably simple answers to this. At some fundamental level, birds are really good indicators of their environment and the health of their environment. Because of that, studying migratory birds in particular, because they connect so many parts of the planet, is just a very good way of starting to quantify and qualify, how are our ecosystems? How do we value them? How do we evaluate them? How healthy are they?
It’s also a very good way to engage people.
And the third piece has to do with basic science. Understanding the patterns and observations that we can make as an observer with binoculars or ears to the sky is as important and increasingly connected to everything we can learn from the technology we have. It wasn’t until 2018 that we had a number on how many birds are migrating at night over the U.S. That’s a pretty fundamental quantity that you want to know.
Malena: How is artificial intelligence and machine learning used in BirdCast?
Andrew: We need to, from the radar data, extract what’s weather and what’s biology or birds. Labeling that and turning it into a model that then can very quickly assign, “oh, we’re talking only about birds in these data,” machine learning is critical for that. And it’s a great opportunity to do machine learning because there are a lot of data [and] we can label those data. The heaviest lift for machine learning in the project relates to that creation of the datasets, talking about bird migration as opposed to precipitation or other atmospheric phenomena.
There are other interesting, peripheral places where machine learning is starting to be involved, and probably will be increasingly more so, like the integration of additional data. Radar is great at telling you numbers of birds and patterns of big scales, but it can’t identify the species. The integration of what we call citizen science or community science, basically the bird observations … or flight calls and vocalizations of birds migrating at night … is going to be increasingly a machine learning-driven endeavor. And it’s going to require a lot of computation, but [it’s] something that I think is well within reach in the coming years.
Malena: How accurate is it?
Andrew: The general pattern of what’s birds and what’s not, the model … does really well with that, like way high 90s if you were to try to assign a percentage, some sort of correctness. And we still need to train it further. There always are interesting new cases, but they’re increasingly rare.
The forecast model is different because the forecast model’s relating that dataset (bird migration on radar) to something else (weather variables). That model also behaves really well [at about 80 percent accuracy]. It’s not perfect, as 80 percent is not 100 percent. But it is by far more than good enough to do a great job of predicting bird migration intensity across the U.S. where we’ve employed this, and to do so consistently across the years.
Malena: When you’re training these algorithms on how to pick out birds from everything else, what sort of things are you telling it to look for?
Andrew: There are some really simple patterns that highlight first, “okay, this is meteorological and this is not.” The meteorology that you often see on radar is really irregular patterns of rain—irregular in terms of the shapes that appear in the radar images that are not uniform. They also tend to have these pixel values, as well, describing the reflectivity, or the amount of energy that is scattered back to the radar that are super variable, like thunderstorms—really high intensity, very localized.
And that is a very different pattern from the bird migration signal on radar, and frankly, insects as well. The biology pattern [tends] to be very uniform and doesn’t have that wildly variable or irregular shape. When you’re labeling these data as an expert … [you can say] “given what I know about bird migration and also the wavelength of the radar that birds are way more likely to be detected than insects, I’m going to label this as bird migration. And oh, yeah, by the way, I know what the winds are. And there’s another product of data that comes from the radar about the velocity of the things that are in the atmosphere relative to the radar. Oh, and these things are moving against that—these are definitely birds.”
Again, it’s not perfect. Despite the wavelength issue and that birds are way more likely to be detected, there are cases where there are very, very large numbers of insects that are large and fast flying. So there are these places where we just don’t know, “is that birds or insects? Not sure. I know it’s biological; it’s not meteorological.” So you label it that way. We haven’t used those data so much in the model. But in the future, we will. It’ll grow into something valuable once that dataset grows. That’s another future thing.
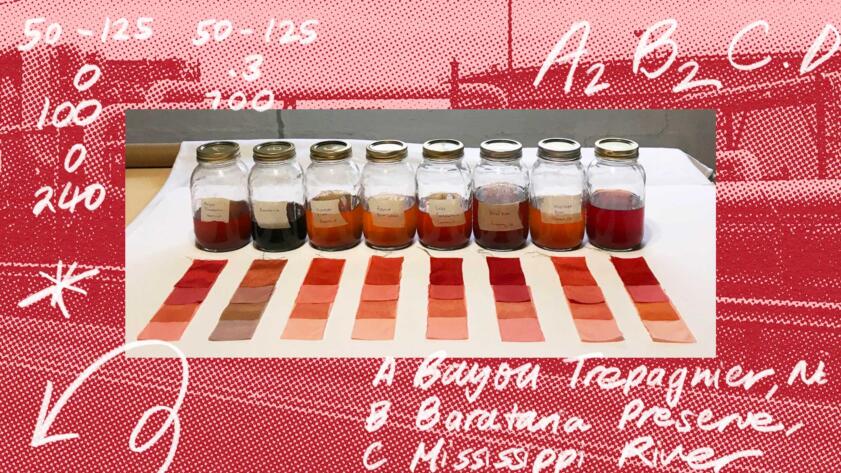
Hello World
The Color of Data
Dyeing fabric might seem like the farthest thing from data journalism, but one artist used it to try to quantify pollution in her community
Malena: You mentioned a few things already, but what can’t AI do yet for BirdCast that would make it better? And how close are you to achieving that?
Andrew: There’s a lot more information in the radar data at the rawest sense, like right off of the radar station, that as biologists we don’t usually get to see. It gets filtered, it gets adjusted right away from a meteorological perspective. [AI could potentially] resolve additional patterns and also having models that take advantage of the different moments that radar produces.
At a simpler level, there’s a real opportunity to have the current machine learning model do even better when it comes to in particular…the birds, insects, bats interface of “well I know this is biological, but I’m not sure what.”
Malena: More generally, how is AI changing the field of ornithology?
Andrew: It’s changing it in pretty huge ways. From a general perspective, the idea that we can now use these kinds of models and this kind of computational power to recognize images and sounds … and do so with super high confidence, that’s huge. People may not realize that—they may think, “I’m out birding, I’m not engaging with technology so much. Yeah I’ll use my phone as my ID guide, and I’ll have it answer some questions for me if I don’t know the answers.” But that means direct engagement with machine learning every time you do that. So I think there’s a huge, huge connection very broadly to how we do observations.
Malena: Ornithology is one of the few areas of science where regular people can meaningfully contribute. What role do regular citizens play in ornithology where AI is concerned?
Andrew: I think that there actually is a really important role. Humans are important in AI modeling and all machine learning in that they are the experts. All the things you do in your brain that gets you from, “Oh, I saw a robin, and I saw 10 of them, and they were at this particular spot.” That’s essential information for these models going forward. And so the contributions become the raw data … the machine learning operates on. And there are other places where going out and being an observer on the ground where the radar data are sampling something in the atmosphere and being able to make that connection, these are important to that kind of ground truthing. The information that the humans produce, whatever it is, you know, whether you’re an expert, or whether you are a novice, can be really essential as like kernels of what these machine learning models operate on.
Thanks for reading.
Sincerely,
Malena Carollo
Investigative Reporter
The Markup