Subscribe to Hello World
Hello World is a weekly newsletter—delivered every Saturday morning—that goes deep into our original reporting and the questions we put to big thinkers in the field. Browse the archive here.
Hi! I’m Natasha, the new statistical journalist here at The Markup.
My journey into journalism has not been a linear one; before joining a newsroom, I spent several years as a statistician in academia. But my goal throughout my career has remained the same: challenge technology to serve the public good, as The Markup’s mission states. Finding positions where I have the opportunity to do so? That’s been a whole other challenge (at least for my idea of public good).
When I was fresh out of college, my plan was simple: score an awesome job where I could use machine learning to address climate change and its consequences. But the college-to-career recruiting pipeline kept dumping me on the doorsteps of big tech companies, and my own efforts weren’t getting me much further. There was a sea of opportunities to do things like improve ad-targeting algorithms, advance self-driving cars, or model human decision-making with a computer program, but shockingly little in anything related to climate. It was this tedious and often frustrating search that introduced me to a handful of organizations that stood out for pioneering how to use AI to protect the environment.
If you haven’t been down a similar rabbit hole, you may not have heard of Rainforest Connection, a nonprofit that employs AI to help preserve the world’s rainforests. Rainforest Connection’s core idea is simple but ingenious: listen (literally) to the rainforest. The organization collects sound from rainforests in 37 countries across the globe, recording audio from what equates to nearly 2 million acres of land, much of it in remote corners of dense jungle. Rainforest Connection’s AI tools parse this data to do things like monitor biodiversity and detect instances of illegal deforestation or animal poaching. But their work doesn’t just take place behind a computer screen; Rainforest Connection collaborates with local organizations and Indigenous communities to guide conservation efforts and facilitate real-time interventions to stop illegal activities.
I spoke with Bourhan Yassin, Rainforest Connection’s CEO, to discuss the role of AI in conservation, how his organization works to bridge the knowledge gap between scientific institutions and the public, and why AI on its own is not enough to generate real-world impact. Our conversation has been edited for brevity and clarity.
Natasha Uzcátegui-Liggett: Can you tell me a little bit more about the work that Rainforest Connection does?
Bourhan Yassin: Rainforest Connection uses sound as a way to understand what’s going on in forests and other ecosystems. Sound is a really good way of being able to detect things from a long distance, and it works in a 360-degree fashion. We use sound in a couple of different ways. One, we use sound to detect illegal activities. Sound in combination with AI can detect things like chainsaws, which are indicative of illegal logging, or gunshots, which are indicative of poaching.
We also use sound to do what’s called “biodiversity monitoring.” We’re able to use AI to detect individual types of species and even the type of song or vocalization. A lot of indicator species, species that indicate the health of the forest, are vocal, like birds and amphibians, so monitoring their sounds also allows us to measure the health of the forest. The success of many projects, whether it’s a restoration project or a project to create a protected wildlife area, depends on showing whether biodiversity is improving because, ultimately, that’s the essence of what you’re trying to do. Using a combination of AI, machine learning, and a bunch of tools that we’ve developed, we’re able to use sound to track this.
Uzcátegui-Liggett: One of Rainforest Connection’s tools that I’m particularly interested in is the open-source AI platform Arbimon, which can be used by scientists and conservation organizations to analyze their field recordings. How does Arbimon work, and what inspired Rainforest Connection to develop it?
Yassin: Arbimon actually preceded Rainforest Connection. It was born as a research project out of the University of Puerto Rico and was originally designed as a solution for bioacousticians to use technology to facilitate their work. When Rainforest Connection took over in 2019 or so, our aim was to bridge the gap between science and conservation and to drive more conservation action on the ground.
For most people in the scientific community, it seems like publishing a paper is their sort of nirvana moment, but translating that into conservation action is often difficult. The idea behind Arbimon was to provide tools for scientists to fast-track their research, allowing them to get to the point of making discoveries more quickly while also providing a tool for on-the-ground conservation organizations, enabling them to decipher the scientific findings pretty easily.
Our approach is that our science team, who happens to probably be the biggest collection of bioacoustic scientists in the world, takes on direct projects that we receive funding for. Through these projects, we learn different methodologies and techniques, which we ultimately develop into a software solution or AI application that is then made available for free to everybody through Arbimon. For example, we published a paper on a pattern-matching technique several years ago. This was then translated into an Arbimon tool, which has been used to run somewhere around 1.2 billion analyses. We did something similar with cluster analysis, which is a way of grouping sounds.
Uzcátegui-Liggett: Are there challenges for scientists using the Arbimon tool who are not necessarily experienced with or knowledgeable about machine learning?
Yassin: We try to use the no-code approach as much as possible, but you have to figure out a balance between how to make it really easy and how to get to a point where it’s not so easy that it loses its scientific value. The scientific community is quite diverse in terms of its technical capabilities. You have people that have zero technical capabilities, and they just want the ability to analyze audio. And then you have people who can develop an entire model. We certainly serve the first group more at the moment, but we’re interested in finding a way to serve the other group as well by giving them the opportunity to design tools and models and import them.
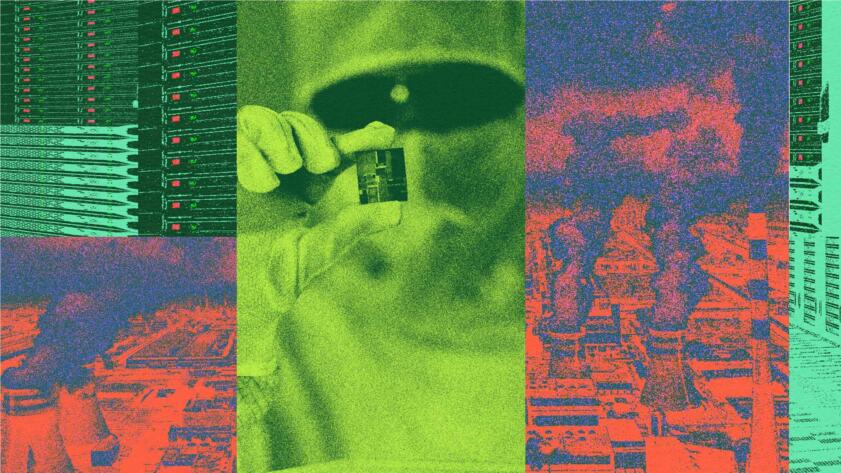
Hello World
AI Environmental Equity: “It’s Not Easy Being Green”
A conversation with Shaolei Ren
Uzcátegui-Liggett: Before I joined The Markup, I spent several years in scientific research at the intersection of climate and machine learning. Considering climate change is one of the biggest issues our world faces, I was shocked at how relatively little research was being conducted in this field in comparison to other AI applications. How has AI helped your organization address the consequences of climate change? What is the future of AI in this space?
Yassin: “AI” is such a broad term, as you know, so let’s talk about AI for detecting patterns. A human would normally take anywhere between 10 to 15 minutes to process a 60-second audio file because they have to listen to it multiple times, make notes on what the species is and when they heard it, etc. AI can offer automated pattern detection at an enormous scale, taking ridiculously large amounts of data and bringing it down to exactly what is important.
We went from active acoustic monitoring, where scientists would hide behind a bush in the forest, pointing a sharp mic at an animal to record it, to passive monitoring, where we can record the entire soundscape, 360 degrees, for weeks and months at time. For a project we did with the U.S. Fish and Wildlife Service in Puerto Rico, we collected 8 million recordings in a matter of three months. It’s almost impossible for a human to discover the variety of species and their occurrences in that large of a dataset. AI and machine learning is absolutely critical in enabling this way of detecting patterns and is able to do this very quickly. And it’s not only about automating the job. AI is able to detect these patterns better than the human ear; it’s trained over so many examples that it’s able to adapt better.
Another important piece is quantitative ecology. Now that we have all this information on the occurrences of species, how do you compare that to, for example, land cover or precipitation or other datasets that offer you a glimpse of what’s going on in the forest? That’s where AI is important; you can start to see those trends. In Puerto Rico, we saw that animals are moving to higher elevations because Puerto Rico is getting drier and drier. Discovering these trends allows us to make recommendations for what needs to change on the ground in order to adapt for that.
I think the biggest learning has been that technology is useless on its own.
Bourhan Yassin
Uzcátegui-Liggett: Rainforest Connection has been conducting research for over a decade. What have you learned in that time?
Yassin: I think the biggest learning has been that technology is useless on its own. When you’re a nonprofit, you care about impact, and impact is dependent on whether the product is being used. One example that comes to mind is our work with the Tembé Indigenous tribe from Brazil. We provided them with a system to detect illegal logging—and although the technology was working as intended, we saw that they wouldn’t respond to the alerts very often, they wouldn’t follow the process. What we learned as we continued to work with them was that they needed a better support structure on the ground. They needed funding to fix their bikes, for example, so they could go on patrols. Once we started providing assistance around the organizational aspects, the technology became very effective. Technology alone is not enough. It’s technology along with figuring out how people would use it, especially if you’re working with people who don’t have lots of resources.
Uzcátegui-Liggett: Historically, Indigenous communities have been excluded from decision-making around AI. Could you talk about how your organization approaches collaborating with Indigenous communities on AI solutions? What are the considerations when integrating this technology into vastly different communities around the world?
Yassin: We’ve struggled with that quite a bit. It’s quite challenging to develop a common AI tool that works, for example, both for groups of villages in Sumatra, Indonesia, and also for the Tembé tribe in Brazil. There are so many differences between the two. One of the biggest decisions Rainforest Connection made early on, which has proven to be quite fruitful, was to be on the ground with people. We’re the ones climbing the trees to do the installation, we do the training, we send in biodiversity scientists and hardware experts. We embed ourselves with the people, and that gives us an opportunity to get an unfiltered channel of feedback on what works and what doesn’t. Just yesterday, I had a call with our field manager who was in the Congo. We often have debriefs to talk about what we learned, what went wrong, what went well, and how we can make improvements to the hardware, software, and the AI tools.
It’s very important, especially for communities and people who are routinely overlooked, to listen to them and understand what their requirements are. It’s almost impossible to do that remotely. Maintaining that connection with our partners is so important to us.
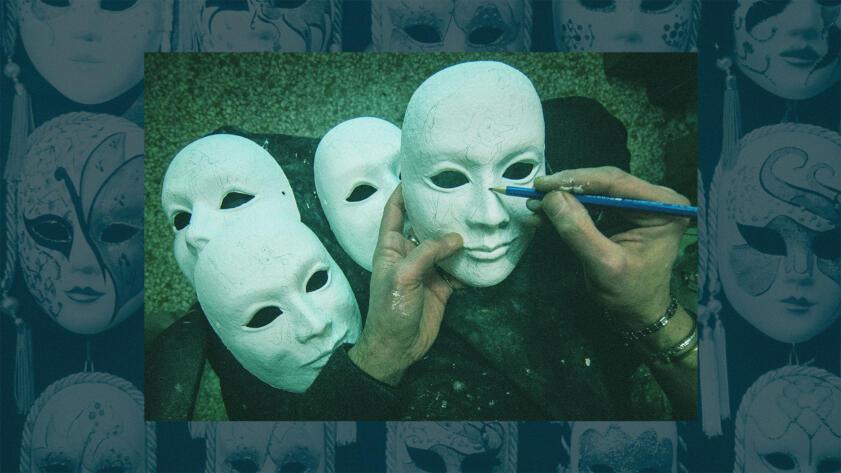
Hello World
‘Unmasking AI’ and the Fight for Algorithmic Justice
A conversation with Dr. Joy Buolamwini
Uzcátegui-Liggett: Scientific findings often aren’t effectively communicated to the communities and causes they are meant to serve. What does your organization do to help bridge this gap, especially as your analyses are becoming increasingly complex with advancements in machine learning?
Yassin: I almost wish there were a mandate that essentially encourages researchers to publish papers that actually have an application on the ground. I think that would go a long way. The paper itself is sort of the focus, the place where researchers achieve their mission, so to speak. Sometimes the more complicated it is, the more technical jargon it contains, perhaps the better it is for certain researchers, but that leaves the people on the ground behind, which is unfortunate.
Our team publishes papers in line with how other research papers are, so they’re certainly not the most useful on the ground. But if we can turn that into a tool, where someone can click a couple of buttons and get the exact results that we spent a year and a whole paper developing, that’s our way of sort of bridging the gap. Instead of trying to find an easier way to describe something so complex, we create a tool for you that you can use in a simple manner.
This is the main purpose of Arbimon. Take pattern matching, for example, the methodology of discovering patterns in audio to facilitate data labeling. If you’re a researcher or a scientist, you can read publications about it, you can look at the code that has been published. But if you’re a conservation organization, you won’t know where to start. In Arbimon, you can drag and drop your audio, specify which pattern you’re looking for, and hit go. So for us, turning our findings into a tool has been the best way to bridge that gap.
We have, I would say, the biggest collection of soundscape data that ever existed, and it is quite underutilized on a global level at the moment.
Bourhan Yassin
Uzcátegui-Liggett: At The Markup, we think a lot about the harms of AI-facilitated mass surveillance in the human world. I feel like I have an almost reflexive, cautionary reaction to any type of surveillance. The harms of surveillance that we see in the human world don’t appear to be a factor in the work that you do, but can you foresee any consequences of this type of surveillance in the natural world?
Yassin: Well, the biggest consequences I have ever thought about, and the reason why we turn down so many real-world applications of our technology, is having it used for things that don’t coincide with our mission. For example, we’ve had mining companies come to us and say, Hey, we’d love to use your technology to monitor how we’re rebuilding after we’ve destroyed this entire area. O.K., no thanks. We’re good. Or another example is using it to detect disturbances in human applications. That’s not what it was intended to do.
Uzcátegui-Liggett: What’s next for Rainforest Connection?
Yassin: That’s always a good question. There are so many things. We want to make good use of all this data we have. We have, I would say, the biggest collection of soundscape data that ever existed, and it is quite underutilized on a global level at the moment. We’re interested in evolving beyond our individual projects to pursue global level analytics that allow us to understand the breadth of our data and what the state of the natural world looks like at a higher level.
At The Markup, I’m interested in covering the intersection of technology and climate and conservation and the ways AI plays a role in environmental inequity. Have ideas on what I should be looking into? I’d love to hear from you! You can reach me at natasha@themarkup.org or (720) 772-1576.
Till next time,
Natasha Uzcátegui-Liggett
Statistical Journalist
The Markup