Subscribe to Hello World
Hello World is a weekly newsletter—delivered every Saturday morning—that goes deep into our original reporting and the questions we put to big thinkers in the field. Browse the archive here.
Hi everyone,
It’s Ross. I’m back with some more deep discussion of AI policy. This week, I’m catching up with Jonathan Frankle, Chief Scientist at DataBricks, a data storage and processing platform. Frankle served as the inaugural Staff Technologist at the Center on Privacy and Technology at Georgetown Law, where he worked on police use of facial recognition and co-developed a course on Computer Programming for Lawyers. After obtaining his PhD, Frankle launched MosaicML, a startup that allowed companies to train LLMs on their own data, which was then acquired by DataBricks last year. He continues to work on AI policy issues, currently with the Organization for Economic Cooperation and Development (OECD), whose AI Principles influence policy around the world.
I sat down (virtually) with Jonathan Frankle to discuss the ethics of companies using customer data to train models, the growing trend of integrating AI models into our personal devices and lives, and how people can get involved in policy conversations from national to local level. Our conversation has been edited for brevity and clarity.
Ross Teixeira: A lot of your work at Databricks is focused around helping customers make the most of the data they have. Facebook and Adobe recently tried to start training generative AI models on their user’s data and got a lot of pushback from that. Is there overlap with that and the work you do at Databricks?
Jonathan Frankle: The nice thing about my role is, we don’t use any customer data for training models. The customer’s data is the customer’s data. That is, like, the most sacred thing in the world. The only places where we’ve even talked about that—and this is actually one of the cooler things I’m more excited about—is inviting our customers to share their evaluation datasets with us for internal use. (Evaluation datasets are example behaviors that a model should follow used to measure how well it is performing.) The science of fine-tuning models is really messy, and a lot of our customers have said, with appropriate security safeguards and appropriate care, “We’ll happily share our evaluation sets with you.”
What about our customers and how they use their customer’s data? For a lot of these use cases, it’s bringing new technology to solve existing problems. Our customers are already using machine learning-driven systems—think fraud detection banking. Wouldn’t it be nice if you could use large language models to try to help explain these fraud detection graphs that show up to a human, so that someone can try to make sense of them right away? I’ve seen very few new use cases from our customers.
In a “contextual integrity” view of the world, privacy violations are those in which data is collected in one context and used in another context, especially a context that was not predictable or not foreseeable. So when I look at what our customers are doing, for the most part, user data is used within context, but it’s used to take advantage of newer, fancier, better technology to solve their existing problems or to augment some existing process.
Teixeira: Recently, everyone was thinking Apple was going to roll out their own LLM integration in their recent iOS, and they ended up announcing that they’re going to roll out ChatGPT integration instead. I’m curious if you have thoughts on what might have led to that decision, or what you think this means for the recent trend of personal devices hosting smaller, local, and private AI models.
Frankle: I love questions about running AI on personal devices because of trade-offs like: Is your device powerful enough? Do you have access to an unlimited data plan, which is not the case for the majority of mobile users around the world?
Then you get into privacy questions, which involves not just “where is the data” and “what is the data being used for,” but also “how do people feel about it?” Dan Solove, a leading expert in privacy law, proposed that the mere fact that you feel like you’re being watched is a privacy violation that is impinging on your freedom and your sense that you can speak your mind or take actions. So there are lots of reasons why continuing to compress these models and continuing to get them on devices makes a ton of sense.
Updating these models gets really tricky because these are gonna be huge models in terms of storage size, even if they’re very small in terms of compute footprint. So there are technical aspects, there are privacy aspects, and there are user comfort aspects.
Teixeira: There’s a big distinction between technical work and policy work, which is very value-driven and needs to understand people. How do you reconcile those two things when you think about how technical knowledge can apply to the policy world?
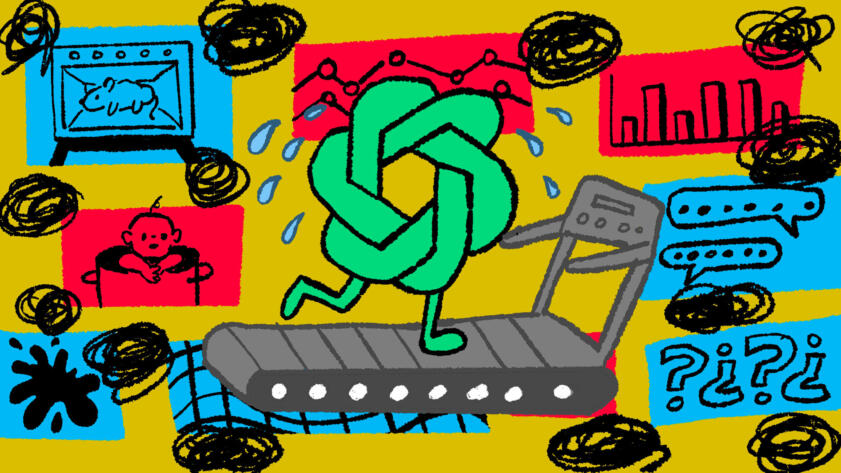
Artificial Intelligence
Everyone Is Judging AI by These Tests. But Experts Say They’re Close to Meaningless
Benchmarks used to rank AI models are several years old, often sourced from amateur websites, and, experts worry, lending automated systems a dubious sense of authority
Frankle: I think a lot of times when you get very value driven, you get driven by the world you want to see and sometimes lose a bit of touch with the world as it is, or even how policy has been able to successfully or unsuccessfully affect the world.
What I’ve loved about the OECD, just speaking personally, has been they are data-driven. Like, the whole point is they’re a bunch of economists, and economists are boring sometimes, but boring in the best possible way. They love their data. They love their measurement. The OECD has an addiction to trying to measure things. and then trying to use those measurements to inform policy. It’s hard to come up with good measures, but they do a pretty darn good job given how hard it is to measure anything.
I think it is hard for people who come from a technical background to accept that there’s not going to be a right answer. And it’s not going to be measurable. And there’s no objective truth, in some sense of policy. But it is about trying to do your best and do your best towards serving whatever your goals are. Ideally, serving society.
Teixeira: Can you capture people’s feelings and experiences in a data-oriented way?
Frankle: Privacy studies, in particular, have been so much fun to watch over the past few years because you have what people will say in a survey, and then you can go and measure how they actually use their device. And all these amazing surveys show that privacy is the first thing people are willing to give up. There was a study where students were offered pizza if they shared their friends’ email addresses, and a bunch of students did.
Maybe that says people’s preferences are wrong, and they’re just saying one thing and really they don’t care. Maybe that says that we’ve created a world that’s structured such that it’s really hard for people to take actions that are in line with their preferences. Either way, that’s a policy consideration. But such surveys give us the data to make good decisions.
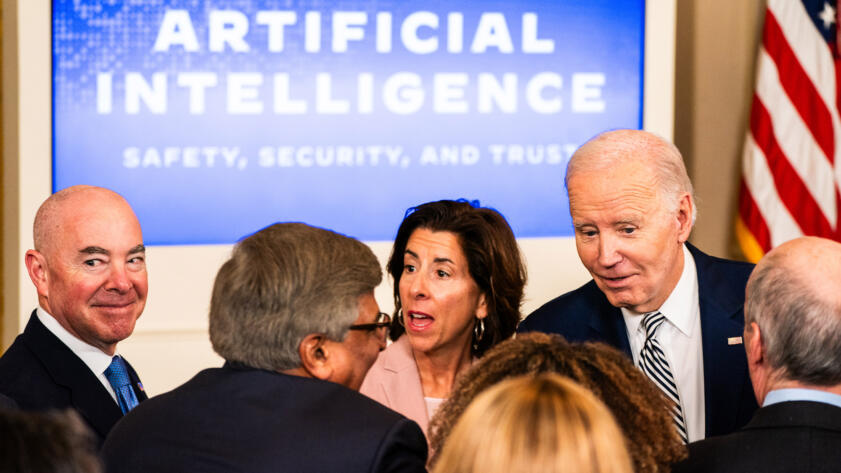
Hello World
Why Governments Need To Take a More Active Role in Regulating AI
Christelle Tessono talks with the Markup about how AI systems are still mostly monitored by the companies that build them
Teixeira: OECD is an international organization, and views about privacy differ greatly between different countries. The United States has a very different sense of what data they want private from other people versus the government, versus Europe or Asia. Do the differences in cultures impact any of any of those policy discussions?
Frankle: Oh, they definitely do. It’s really, really satisfying to have those conversations. Hearing very different viewpoints among how we’ve done regulation, such as the sectoral approach in the U.S., with regulation carefully designed for specific areas like transportation or finance, versus the more general approach in the EU, and getting to have conversations about how’s that working on both sides.
One example that sticks in my mind is, there was an EU policy figure who said they really wanted to start looking at AI and fake news and democracy. This was relatively early on when these were not exactly the hot questions. I remember wondering, “Why democracy?” Well, countries in the EU have quite recent experiences of what it’s like to not live under democracy, and I can see how that informs a lot of questions around privacy, surveillance, and misinformation in the EU. And perhaps it should inform those questions in the US, as well.
Teixeira: Did those conversations ever carry back to your technical work? Was there technical work you changed direction on or put a pause on as a result of being involved in these policy discussions?
Frankle: I have never been good at interdisciplinary work. So in my work, I try to get as deep technically as I can, and always keep in mind how I can share what I’ve learned from my technical experience that matters for a policy audience.
Looking back over the past 18 months, I think LLMs have gotten faster and cheaper, but they haven’t really gotten better.
Jonathan Frankle
There’s certainly conversations we have around DataBricks among my team about what we should worry or care about. By putting a technology into the world, you can make certain things easier, and that doesn’t always work out in a way that is evenly balanced between good and bad, or intrinsically balanced towards good.
Some people are worried for reasons that I’m not as concerned about, such as, “Could AI tell you how to create a novel bioweapon?” Personally, I’m worried about the more mundane, boring stuff. You can fine-tune a model for all sorts of purposes that I think would make a lot of us uncomfortable, such as law enforcement’s use of advanced biometrics that make it easy to track people. And that’s something that really eats me up.
Teixeira: In the long-term process of policy, what do you think about the divide between people who say there’s an imminent risk of AI taking over the world, and those who are skeptical of it?
Frankle: I think with AI, the tricky part for long-term questions is not just that we’re thinking about the long term, but that we have to make assumptions about the nature of how the technology will evolve. And that is an incredibly fraught topic in any policy domain.
My personal take is that technology doesn’t move in this nice linear way that follows scaling laws. Technology tends to have these big bursts of progress, and then a lot of consolidation. Looking back over the past 18 months, I think LLMs have gotten faster and cheaper, but they haven’t really gotten better.
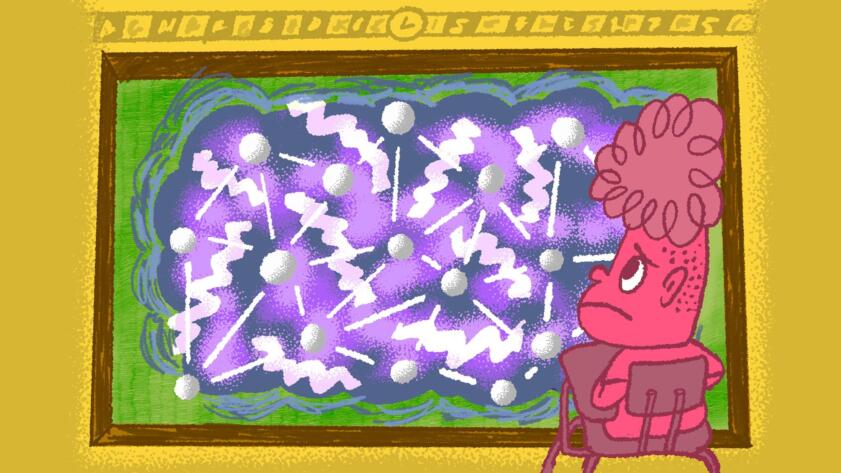
Artificial Intelligence
California’s Two Biggest School Districts Botched AI deals. Here Are Lessons From Their Mistakes
Educators can learn caution from AI mistakes in Los Angeles and San Diego. But they also face pressure to adopt the technology quickly
But assuming that technology froze today and GPT-4 was as good as it got, there’s still gonna be decades of innovation on top of that with unimaginably cool and scary things just based on that one capability, in the same way that internet architecture basically froze in the ’90s. In some sense, the fundamental technology hasn’t changed in decades. The same could be true in AI, and we’ll still have our hands full from a policy perspective.
Teixeira: One of The Markup’s goals is to help readers restore their agency over technology. Do you have any tips for people that want to get more involved in policy but don’t know how to make their voice heard?
Frankle: In terms of how you get in, there are policy conversations happening everywhere. The nice thing about these policy questions is they are not just national or international. Questions of law enforcement use of facial recognition are actually exclusively local, in a sense. They’re the stuff of city council meetings. Your city council has a meeting. Go to it! Go speak!
It doesn’t have to be big things. When you’re at that smaller meeting, you may literally be the only one who shows up to speak about it, and you can have an outsized impact. That can sometimes be a scary thing, but that’s also a really empowering thing.
Have you gotten involved with technology policy affecting your community? If so, please get in touch! For more on AI, read what happened when two California school districts adopted AI tools, or my discussion with Christelle Tessono of the role of government in regulating AI.
Thanks for reading,
Ross Teixeira
Investigative Data Journalist
The Markup / CalMatters