Subscribe to Hello World
Hello World is a weekly newsletter—delivered every Saturday morning—that goes deep into our original reporting and the questions we put to big thinkers in the field. Browse the archive here.
Hi everyone,
Happy New Year. It’s Sisi here.
In 2023, many companies started hiring for an AI prompt engineer. The skill of “prompt engineering” is essentially how well someone can write instructions for large language models (LLMs) such as ChatGPT, in order to get the most useful results.
There are now mountains of guides on how to write the best prompts online, and a commonly used suggestion is that users should assign the LLM a role—“You are a thoughtful manager” or “You are an experienced fundraiser,” for example—before giving the LLM instructions on what to do.
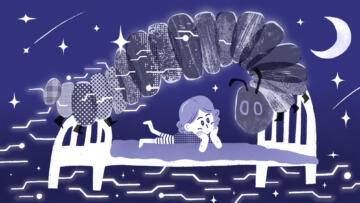
Hello World
The Very Hungry Algorithm: Bedtime with ChatGPT
Balancing enthusiasm and fear in the age of AI
But the idea of LLMs assuming a role also poses some really interesting questions. How do these models interpret being a “doctor,” a “parent,” or even a “psychological profiling AI”?
A team at the University of Michigan recently published a paper that systematically explored how effective giving LLMs a role can be, and how the role you assign can change the accuracy of the answers you get. Their findings had some very interesting surprises. For example, they found that assigning LLMs a role that should be more experienced in a domain area doesn’t result in more accurate responses.
But in addition to looking at professional occupations (“banker”), they also analyzed six other types of roles, including roles related to personal relationships: family (“daughter”), school (“instructor”), romantic (“wife”), work (“boss”), social (“buddy”), and types of AI (“Medical Diagnostic AI”). They then tested the accuracy of these roles by asking the LLMs a multiple-choice question.
So, what did they find? Here’s my Q&A with the team at the University of Michigan—research assistant Mingqian Zheng, Ph.D candidate Jiaxin Pei, and David Jurgens, associate professor in the school of information, and department of computer science and engineering—on their work with collaborators from LG AI, Moontae Lee and Lajanugen Logeswaran.
Our interview has been edited for brevity and clarity.
Sisi: What prompted you to research how effective it would be to assign interpersonal roles like mother, husband, or best friend to LLMs?
David: In the past, you needed special computer knowledge to work with advanced AI but now people can just have a conversation. LLMs like ChatGPT are trained to respond in conversation and from the perspectives of different attitudes, skill-levels, or even people. LLM designers use this ability to create “system prompts” that prime the LLM to give good answers—like including “a helpful assistant”—and it can make a difference in performance. Since people are asking models to take on social roles, we wanted to see what effect these might have, and whether we could find systematic behavior to make models even more effective.
Jiaxin: Previously, we also studied how different social roles affect interpersonal communications between humans. So we naturally started to think about whether prompting with different social roles would affect the models’ behaviors. Since the system prompts are used in nearly all commercial AI systems, it could basically affect everyone’s experience interacting with AI like ChatGPT.
Sisi: You found that when LLMs were asked to assume a female role, like “mother,” they gave worse answers than if you assigned them “father” or “parent.” I know this study couldn’t find exactly why, but can you talk us through some examples of the gender-based roles you tested and what you found?
Our experiments showed that there wasn’t any benefit to choosing a role from the topical domain of the question.
David Jurgens
Jiaxin: We tried to create a balanced setting where we control for the role but modify the gender. For example, in the partner setting, we have male roles (e.g. boyfriend, husband), female roles (e.g. girlfriend, wife), and neutral roles (partner, significant other). It was surprising that prompting with gender-neutral roles led to better results than male and female roles.
David: There was some small drop in performance for roles associated with women compared to men, but the difference was small enough that it could be due to chance. However, there was a much bigger difference between gender-neutral roles (parent) than gendered roles (mother, father).
Sisi: You also tested what happens when we ask LLMs to assume a professional role. For example, assigning an LLM the role of “a doctor” will probably get you better answers than “your doctor.” What’s your best guess as to why this happens?
Jiaxin: This is a great question! Honestly speaking, I don’t know. There are so many things that we still don’t know about LLMs. One guess is that “a” typically has a higher frequency than “your” in LLM training data, so maybe sentences involving “a” have more data to draw from.
David: We really don’t know for sure but if I had to guess, I think the setting where the model is asked to “imagine talking to your doctor” is going to evoke a bigger social context which makes answering a question more complicated. The scenario where a model is asked to “imagine you are a doctor” is much simpler (socially, at least) so there’s less context a model needs to reason about to generate an answer.
Sisi: Across your experiments, it looks like asking LLMs to roleplay as someone who studies engineering or computer science repeatedly resulted in worse answers—which feels a little counterintuitive and ironic. What do you make of that?
Jiaxin: It is also very surprising to us. Before running the experiment, we thought there would be a domain knowledge effect. For example, “engineers” should answer engineering questions better than other types of roles. However, it didn’t seem to be true based on our result. There are so many possible reasons why. We need more research to really understand how LLMs work in various situations. Another takeaway is that while LLMs are able to perform various tasks and communicate like human beings in many situations, they are still very different from humans in nature.
David: Our experiments showed that there wasn’t any benefit to choosing a role from the topical domain of the question. Sometimes those roles were right, sometimes they were wrong, but there wasn’t much difference between any of them, statistically speaking. We thought that maybe we could even try to predict the right role for a question—like maybe this question would be best answered by a chef, or a nurse, or a lawyer—but that didn’t work either!
Mingqian: We also tried to figure out why there was (or wasn’t) a difference in multiple ways, including the similarity between the prompt we gave and the question we asked the model, levels of predictive uncertainty in the models, and how frequently the roles themselves appeared in Google’s collection of textual data (a proxy to model the word frequency in training data). But these factors couldn’t fully account for the result.
If you want to see more examples of roles that Jiaxin, Mingqian, and David tested, take a look at page two of their paper. You’ll get to see how roles like “sheriff,” “gardener,” and “enthusiast,” among many others, performed in their tests.
🌱 This month, we’re also publishing one privacy tip every weekday from a Markup staffer who actually follows that advice in their own life. If you’ve always wanted to protect more of your privacy, but have felt overwhelmed by all the information out there, check out our series. We’re calling it Gentle January: The Least Intimidating Privacy Tips from The Markup.
I also know that you, Hello World readers, probably have your own privacy tips for us and for each other. We’d love to hear your advice. If you have a minute, send us your answer to this question: What is one practical piece of advice on how to maintain your privacy that you actually do yourself? You can email us your answer, along with how you’d like to be identified, to tips@themarkup.org. We will highlight some of your tips during the last week or so of Gentle January.
Thanks for reading, and I hope your January is a gentle one.
Sincerely,
Sisi Wei
Editor-in-Chief
The Markup