The Markup, now a part of CalMatters, uses investigative reporting, data analysis, and software engineering to challenge technology to serve the public good. Sign up for Klaxon, a newsletter that delivers our stories and tools directly to your inbox.
Last November, The Bookseller reported Dutch publisher Veen Bosch & Keuning, owned by publishing titan Simon & Schuster, was testing the use of artificial intelligence to help translate several of its books to English.
The news set off a ripple of anxiety among book translators, who wondered in industry news stories if this was the beginning of the end for their profession — or if machines could even do the delicate dance of translating literature.
Nuanxed and other supporters of automated translation say it’ll bring more literature to the masses and more work to translators, who would be tasked with editing a rough translation produced by the automated tools.
It also opens up an avenue of growth for an industry struggling to stay afloat in the digital age. Translator Peter Breum, who has translated for Nuanxed, wrote in an email to The Markup that the introduction of the tools marks an “exciting new era from which there’s no turning back.” Breum added that we “should deal with this fact in a constructive and positive manner.”
Others believe it’s way too soon to get excited. The latest wave of so-called AI tools, like ChatGPT, often produce irrelevant statements out of thin air, raising doubts about whether they can stay faithful to an author’s original text. Even when they can, literary translation is a delicate undertaking, requiring a deft touch to bring metaphors, tones and phrases across cultures.
900
Approximate number of translations produced by Nuanxed, a Swedish book-translation technology startup
Source: Nuanxed
Polishing rough translations can be as difficult as translating a text from scratch, said Sean Bye, a translator who serves as the interim executive director of the American Literary Translators Association. When it comes to rough machine translations, he said, he believes publishers are integrating machines to do poorly what human translators can do well in a bid to devalue the latter group’s work.
Automated literary translation is a relatively young endeavor. Nuanxed has the field nearly to itself. The company has produced more than 900 translations to date, spanning multiple languages; in comparison, a database curated by a nonprofit literary translation press at the University of Rochester counts between 800 and 1400 English fiction and poetry translations published in the U.S. during the same period, though it’s unclear whether any Nuanxed titles are part of that count. The conversations that unfold as automated literary translation spreads may offer an early preview of how AI could transform other creative fields.
Pioneering a New Market
Nuanxed launched “with the goal of making more translated books available to readers,” CEO Robert Casten Carlberg wrote in an email to The Markup.
The company’s homepage puts this mission in industry terms: “Give books the audience they deserve.”
It’s true literary translations get little attention. Recent research on the U.S. literary landscape, for instance, found a tiny fraction of reviews in The New York Times Book Review discuss translated books, and nearly half of the translated books published in the U.S. are ported over from just three languages (French, Spanish and German).
Nuanxed’s translations have spanned “over 60 language combinations for publishing houses in many different countries,” according to Casten Carlberg.
The company has also left itself plenty of room for growth, having focused so far mainly on “commercial fiction” — typically described as more mainstream and plot-focused than “literary fiction,” though the lines can get blurred — along with some nonfiction. Suspense novel “Glen Affric” by Karine Giebel, which Nuanxed translated from French to English for HarperCollins, fits this mold. The book tells a dark story of two brothers “loosely inspired” by John Steinbeck’s “Of Mice and Men.”
Casten Calberg wrote that by Nuanxed expects to create more work for translators by making it more affordable and easy for publishers to translate books.
Author and translator Steve Anderson, who has translated a book for Nuanxed, thinks it is “onto something that could work,” and he’s cautiously optimistic the company and its technology can indeed create more jobs for translators. That’s partly because, as he sees it, Nuanxed’s workflow prioritizes the “human touch” — which his experience tells him will continue to be necessary in literary translation.
Nuanxed’s tools enable translators to spend less time on more straightforward parts of the text and focus instead on parts where the words “mean the most,” Anderson said. The tools also present the source text and automated output in a helpful interface, he said, and using them can cut the time it takes to translate a book from several months to several weeks.
In a 2024 Society of Authors survey, more than a third of translators reported losing work due to generative tools.
But the way automated tools are developed has prompted concerns among translators that their work is used without payment, credit or consent to “train” the technology that threatens their very livelihoods — all while enriching the companies behind it. In a 2024 Society of Authors survey, more than a third of translators reported losing work due to generative tools.
Russell Scott Valentino, a former president of the American Literary Translators Association, understands that publishers’ financial constraints may tempt them to favor machines over humans—especially big publishers, who he says are mostly chasing a bottom line. But to him, those constraints are a poor excuse for “playing fast and loose with people’s work.”
Valentino does see the potential in using automated tools—combined with good editing, he emphasized—to help translate works that often fly under the radar, like those published in small literary presses: “I don’t know if it sounds a little utopian, but it does sound like it could be cool.”
Casten Carlberg and other supporters of automated translation argue it isn’t meant to replace translators and that instead, translators will fill an editing role by polishing AI-translated drafts in a process often referred to as “post-editing.”
But incorporating AI-based automation to translation work comes with caveats whose roots lie in how automated translation has evolved.
The Evolution of Automated Translation
Early automated, or “machine,” translation relied on rules that specified how languages were structured. The rules were handcrafted by linguistic and language experts, said computational linguist and computer scientist Margaret Mitchell, making the approach hard to scale.
As Mitchell explains it, machine translation began to rely more on statistics around the early 2000s—computer programs would process existing translations and draw associations between the original text and the translated version. The models split input texts into smaller pieces and determined the most likely translation for each based on how similar pieces from previously-processed texts were translated.
In the 2010s, the state of the art shifted again to deep learning models that relied on neural networks, or collections of computing “nodes,” an idea initially inspired by the neurons of the human brain. Nodes — AI software instances — perform computations based on other nodes’ parameters that are automatically updated to yield the best results as the network is trained on source and target texts. Given an input text, the model uses those computations to derive the most likely translation.
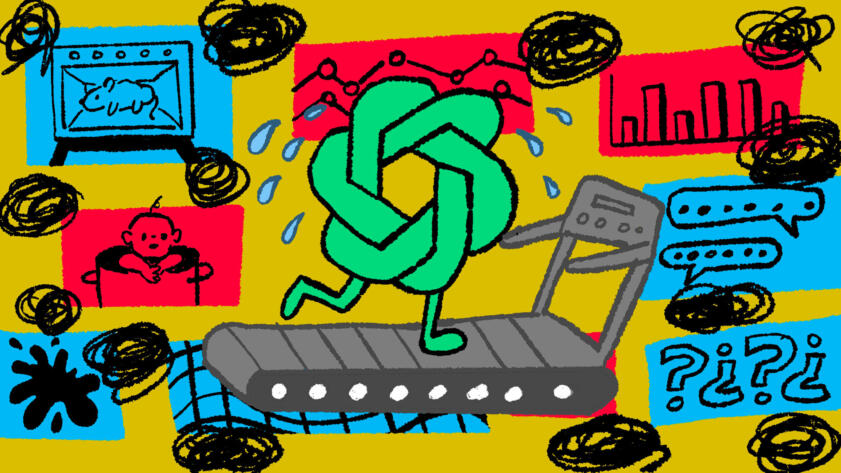
Artificial Intelligence
Everyone Is Judging AI by These Tests. But Experts Say They’re Close to Meaningless
Benchmarks used to rank AI models are several years old, often sourced from amateur websites, and, experts worry, lending automated systems a dubious sense of authority
If statistical machine translation was like filling in a blank based on words that came before, deep learning was processing a novel many times to make data-driven projections about what the characters were saying in a given scene. The approach made machine translation “really shine,” Mitchell said, and companies like Microsoft and Google began implementing their own neural network-based systems.
“Generative” approaches were introduced in the mid-2010s but exploded in popularity more recently, powering ChatGPT’s underlying large language models and Stable Diffusion and igniting debates over cognition, emotion and what constitutes art. LLMs like ChatGPT’s rely on neural networks that use “transformer architectures,” which encode words in the input text in relation to each other and come closer to capturing context than traditional machine translation.
It’s unclear whether the tools Veen Bosch & Keuning is testing are based on generative approaches; the publisher could not be reached for comment. Nuanxed, meanwhile, uses “the latest technology wherever it makes sense to create a better translation experience, including generative approaches,” Casten Carlberg wrote.
LLMs are trained on vastly more data than traditional approaches. The data is supposed to contain enough text across enough languages such that when prompted and provided an input text, the model can find and use source and target texts that co-occur and possibly correspond to generate the most likely translation.
But the Internet’s text data — a major data source for LLMs — is messy, and those correspondences may not be perfect. And generative tools’ propensity to produce irrelevant statements known as “hallucinations” can be taxing for translators working with their output.
Emerging Flaws
Despite advances in automated translation, the technology can still fall short in the context of literature.
Linguist Emily M. Bender said while she would expect machine translation to roughly capture meaning in the context of literature, literary translation is “skilled and artistic work” that goes far beyond “what you would expect to come out of statistical correspondences.” Those correspondences can create problems: Bender gave the example that “Amsterdam” may be improperly translated to “Washington, D.C.” or “London” in English, even if the story takes place in Amsterdam.
Mitchell worries the tools will bungle cross-language nuances, like gender and pronouns, jargon and idioms.
Part of the problem, she said, is that automated tools translate better between languages that are well-represented in language datasets, like English, Mandarin, Spanish and French. They perform a lot worse for languages that are less prominent in the data, like Croatian.
Valentino, the former translators’ association president, works with Croatian as a professor of Slavic and East European languages at Indiana University. In 2023, Valentino experimented with translating a part of acclaimed Bosnian writer Miljenko Jergović’s short story “Učiteljica” from Croatian to English using ChatGPT.
Valentino summarized on his blog that the tool produced a serviceable translation with several awkward phrasings—and one red flag, presenting a “dark-skinned” individual as “Black.” The individual is unlikely to be Black, Valentino wrote, given the story’s setting—likely some part of former Yugoslavia—and the original text more likely implies “dark-skinned” in reference to a Jewish or Romani individual. He added in an interview that the mistake would prompt him to approach the output with heightened distrust.
“Still, I like this translation,” Valentino wrote on his blog, after the experiment. “It’s got issues, but the issues are no bigger than those my students routinely face. If someone turned it in as an assignment, or submitted it as a possible publication, I could work with it. If it functioned as my own first draft, knowing what I know, I could most certainly work with it. It took about twenty seconds to create this draft,” he wrote, though he added: “‘Knowing what I know’ is a key caveat.”
A bad translation is worse than no translation because it’s going to block the way to a good translation being produced.
Liesl Yamaguchi, translator
Bye said he worries automated translation’s struggle with translating less-represented languages means it could reinforce structural inequalities that keep certain voices — particularly from the Global South — from entering the book market. Or, he and others worry, translations from less-represented languages may end up poorly done as the tools fail to tactfully handle cultural subtleties..
“A bad translation is worse than no translation because it’s going to block the way to a good translation being produced,” said U.C. Berkeley French professor Liesl Yamaguchi, who translated Väinö Linna’s classic 1954 World War II novel “The Unknown Soldier” from Finnish to English. “It took the rights, it took the resources and people say, ‘Oh, it exists. It’s accessible. So we don’t need to do a good version.’”
“That effectively kills the work in the target language,” Yamaguchi added. “That’s an extremely cruel and unfortunate thing to do to lesser-translated languages and literatures.”
“The Unknown Soldier” is, arguably, an example of this phenomenon. A previous English translation attempt, dating to the 1950s, was widely panned. “It’s a miracle the translation should be so bad,” Finnish author Juri Nummelin wrote, adding that it was “clumsy with the Finnish idioms.” Yamaguchi’s translation has been better received, with a Glasgow Herald reviewer writing that it “finally does justice to the original” in the wake of the prior, “rickety” translation.
Lesan AI co-founder Asmelash Teka Hadgu, who develops machine translation systems for Amharic, Tigrinya and English, believes that automated translation’s performance disparities are really a resource allocation problem. Lesan, for instance, devotes resources to gathering quality data that Hadgu said enables better performance across the three languages, enlisting the help of native speakers to digitize newspapers and books.
In contrast, big-company, big-data, big-model approaches to automated language-related tasks often rely on the Internet as a major data source. It’s unlikely to be a quick fix: Unbalanced language representation and biased speech persists in the Internet’s text data, according to a 2021 paper by a group of researchers that included Bender and Mitchell.
Translating a Technology to a Workflow
For all the potential problems with the technology, a bigger issue may be with how we use it.
Take, for example, “automation bias,” in which the human is primed or pushed toward the machine’s output. The phenomenon has been observed in health and criminal justice settings; Mitchell said it could make the final translations more closely resemble the automated ones.
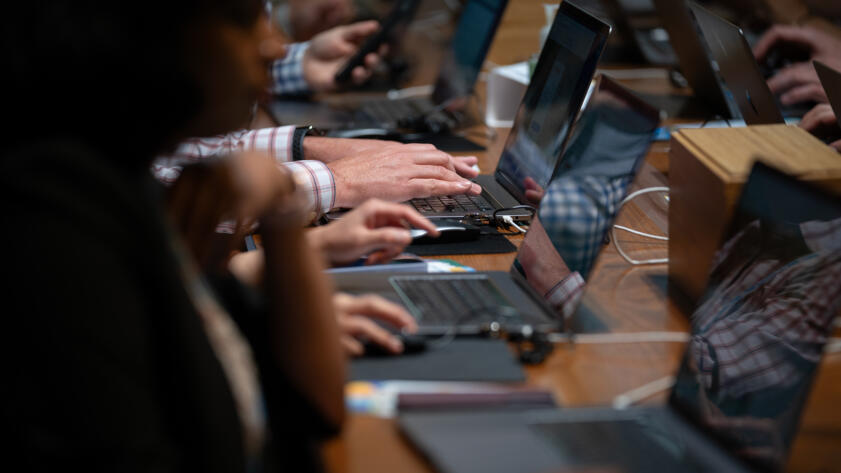
Artificial Intelligence
Fearing AI Will Take Their Jobs, Workers Plan a Long Battle Against Tech
At a landmark gathering in California, workers discussed defenses against artificial intelligence and surveillance technology
Then there’s the question of efficiency, something translation technology was supposed to improve. Some translators note that doing quality post-editing requires tremendous time and care. That’s because automated tools often produce inaccurate but convincing output, forcing translators to “operate with suspicion towards the text,” said Kira Josefsson, a freelancer who organizes for translator labor rights within the National Writers Union.
Bye, who has translated a number of fiction and nonfiction works from Polish to English, characterized literary translation as a subjective process of teasing out the intangible elements that make a book spark its reader’s imagination. Many automated tools are meanwhile marketed as a way to mechanize away some less challenging artistic work. (For instance, Casten Carlberg wrote that Nuanxed’s tools are built “with the aim of removing the heavy lifting” to allow translators, editors and proofreaders to “focus on the creative aspects.”)
Bye believes the attitude misunderstands the nature of literary translation: that the hard part is not in “looking up all of the words that you don’t know and putting them down onto the page” but in “making it sound good.” Because of this, he said, quality post-editing is not merely a matter of skimming and “sprinkling in a little bit of flavor here and there.”
To him, the push for post-editing appears driven not by quality or accessibility goals but by a desire to cut costs. Post-editing pays less than translating, Bye argued, but doesn’t save much time and can sacrifice quality: “Very often you’re better off redoing the whole thing from scratch.”
Very often you’re better off redoing the whole thing from scratch.
Sean Bye, executive director, American Literary Translators Association, on editing automated translations
(Casten Carlberg wrote that the work Nuanxed’s translators do could be called a type of post-editing, but “I’d say it’s more fair to describe it as a combination of editing and translating.”)
Anderson understands why some translators prefer to control the process from beginning to end. He, too, knows post-editing can be a slog if the conditions aren’t right: He’s edited a shoddy automated translation before, without a helpful interface, and agreed it probably took as long as or longer than it would have taken him to do the translation himself.
Adding Risk to a Precarious Occupation
Worries among translators about the labor involved in post-edits go hand-in-hand with broader concerns about working conditions.
Translators were underpaid and largely underrecognized even before automation entered the picture, said linguist, translator and author of “Language City” Ross Perlin. In a 2022 survey of U.S. literary translators by the Authors Guild, nearly two-thirds reported making under $10,000 from literary translations in the prior year. (The median U.S. individual earner made around $37,500 in 2021, according to the Census Bureau.) It wasn’t until 2021 that a push to credit translators on book covers began gaining industry-wide momentum.
$10,000/year
Amount two thirds of literary translators failed to earn from their work in 2022
Source: Authors Guild survey
Breum enjoys post-editing; in his email to The Markup, he wrote that polishing automated output has sharpened his writing and refined his taste. Others find the work undesirable: Translator Ian Giles likened it to the “miserable” task of fixing the output of a “not-very-consistent robot that was drunk.”
Many are already experiencing top-down directives to use automated tools, especially generative ones. The Society of Authors survey found 8 percent of translators reported using generative tools “because their publisher or commissioning organisation asked them to.”
The Authors Guild and the Society of Authors have begun advising translators to include language in their contracts prohibiting publishers from using or selling their work to train LLMs. Bye said he’s skeptical the language will be enforceable, calling for more robust regulation to protect the work of translators and other creative professionals.
Nuanxed has considered some of these issues: It credits its translators, Casten Carlberg wrote, and is “fully transparent with all parties that we build and use technology as tools for translators, editors and proofreaders.” As for whether the company trains its models on its translators’ work, Casten Carlberg wrote that Nuanxed is transparent about “using the work performed to improve the quality of future translations.”
The question of empowerment has taken center stage in the debate over how automation will change an industry grappling more than most with the merciless forces of capitalism. It’s one thing for translators to choose to use automated tools, Perlin said, but it’s another for publishers to “bypass the hard-won expertise of translators just to increase the production of questionable product.” Some worry those who command the levers of power in publishing are veering toward the latter.
Rewriting a Literary Endeavour
Common among critics was a sense that the problem itself had been lost in translation.
Bender likened the argument for automated translation as democratizing agent to an argument for artificial scarcity. “They’re basically saying, ‘Look, we haven’t been doing this,’” and the tools will allow us to do it, she said. “You could do it just by putting more resources into it. It’s not like there aren’t people who can do that work.”
To Giles, too, the better way to bring more literature to the masses is to give translators the support they deserve: “If you think the world needs more translators, maybe the world needs more translators who are human.”
Anderson said he agrees but doesn’t know if “the business demands of the real world will allow for us translators to actually be paid” for this: Publishing is a business at the end of the day, literary translation gigs can be few and far between and many translators do the work as a side hustle, he said. He believes, then, that automation can bring him new opportunities; using Nuanxed, for example, frees up his time to work on other gigs. Because of this, he called the translation versus post-editing pay-rate debate an “apples-to-oranges” comparison.
I, for one, feel fine about not having to beg, haggle and barter in connection with each and every translation.
Peter Breum, translator who has worked with Nuanxed
Breum agreed. While the mental strain of post-editing automated output, which he likened to editing and translating simultaneously, means he can’t work more than six or seven hours a day, Breum wrote, he gets the same amount of work done in five hours of post-editing as ten hours of translating. He claims his pay situation for post-editing is “similar, bordering on better” than for translating due to this speedup.
“I, for one, feel fine about not having to beg, haggle and barter in connection with each and every translation,” Breum added.
Still, Anderson and Breum hope the companies developing automated tools remember that human translators are essential to their operation; that, as Breum wrote, their success “depends on the skills of their editorial employees/post-editors no matter how sophisticated the various platforms may develop into becoming.”
But to Giles, this is impractical. He sees the problem with automated tools as two-fold: Not only will they perform poorly, Giles worries, but they’ll also begin to change our attitudes, normalizing substandard work and setting off a “race to the bottom.”
“If people who currently pay my bills decide that ‘good enough’ is good enough,” he said, “where does that leave me, and where does that leave my colleagues?”
For many, that slip in standards might become the real loss.
In an August 2024 New Yorker essay, “Why A.I. Isn’t Going to Make Art,” science fiction writer Ted Chiang describes the artistic process as a long series of careful choices, automated approximations of it a shorter series of “bland” or “derivative” ones.
To Josefsson, this intentionality is part of “why readers should care.” The nature of statistical tools, operating on what Josefsson likened to a lowest common denominator, removes the “thrill of idiosyncrasy,” the moments of: “‘Wow, what an incredible, surprising turn of phrase,’” she said. “That’s that thing that pierces the humdrum of the world and lends the world specificity.”
So it comes back to the enduring question of why we read literature. Yamaguchi said the answer is simple: We do so for pleasure.
“Typically that pleasure resides in incredible nuances of usage,” Yamaguchi said. “That means it resides in extraordinary translation.”